
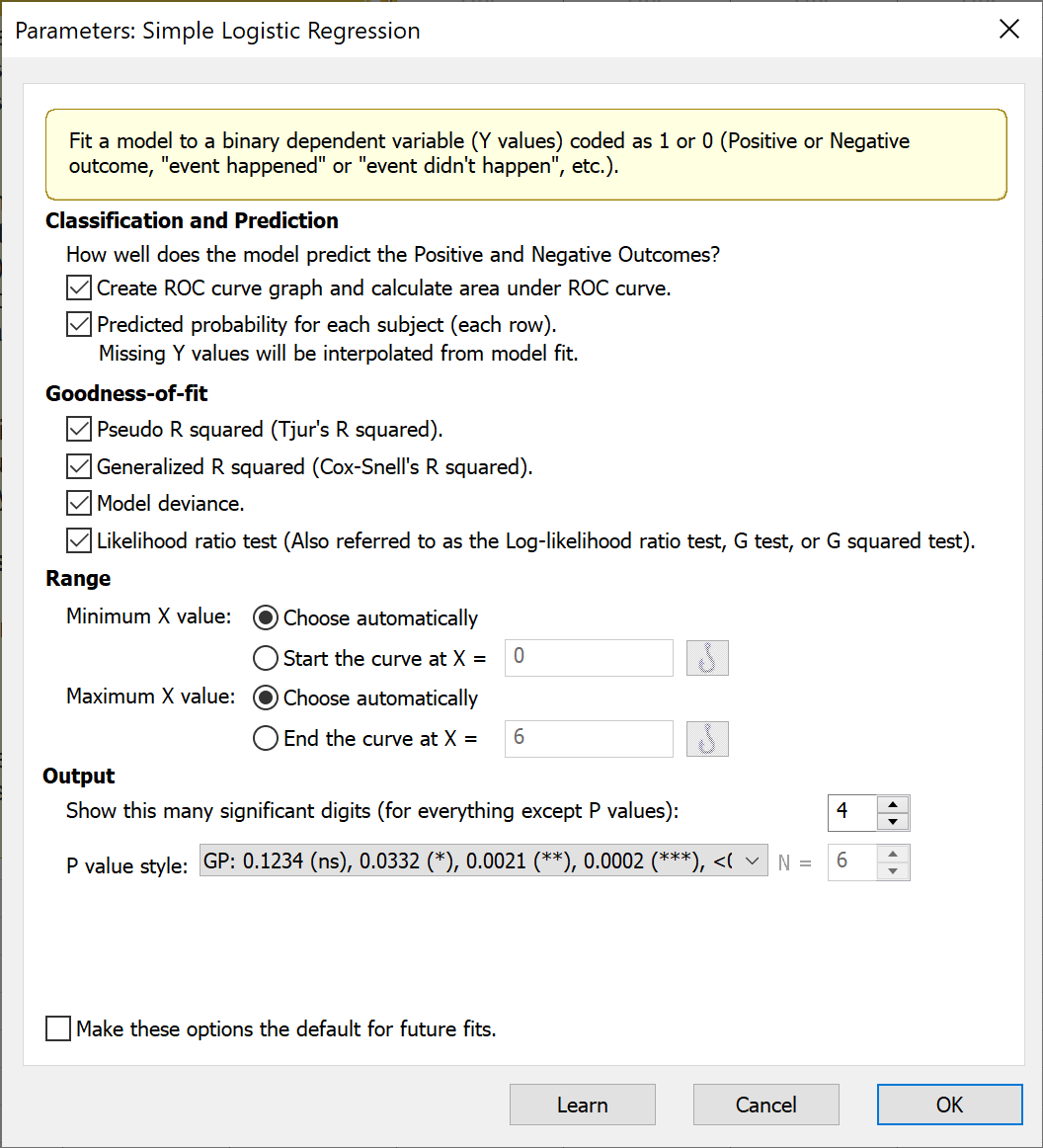
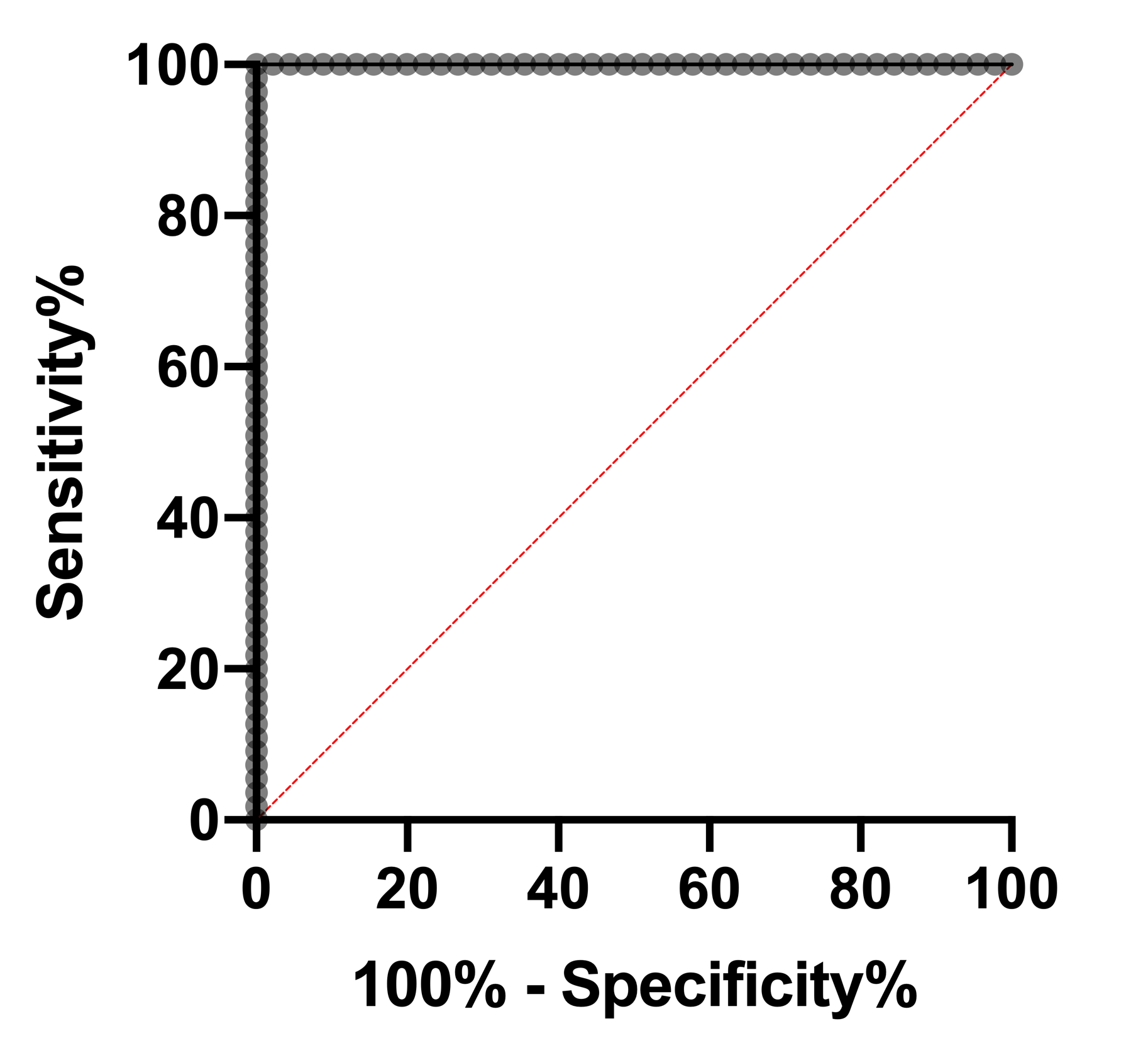
Ĭonsider constraining the parameter HillSlope to its standard values of 1.0. If you prefer to enter concentrations, rather than the logarithm of concentrations, use Prism to transform the X values to logs.įrom the data table, click Analyze, choose nonlinear regression, choose the panel of equations "Dose-response curves - Stimulation" and then choose the equation " log(Agonist) vs. Enter one data set into column A, and use columns B, C. Enter response into Y in any convenient units. Enter the logarithm of the concentration of the agonist into X. It is also called a four-parameter dose-response curve, or four-parameter logistic curve, abbreviated 4PL. This is preferable when you have plenty of data points.
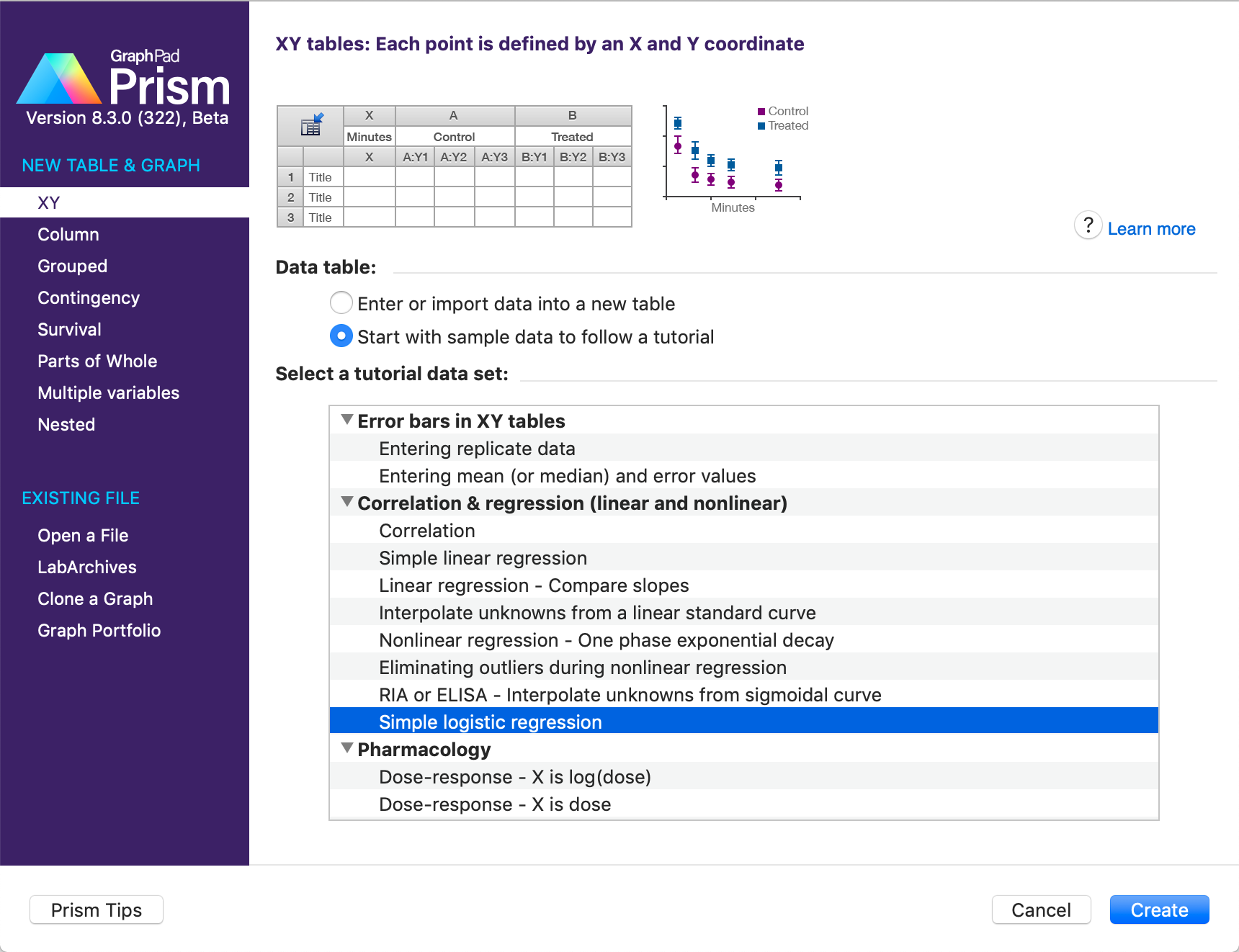
This model does not assume a standard slope but rather fits the Hill Slope from the data, and so is called a Variable slope model. Many dose-response curves have a standard slope of 1.0. The goal is to determine the EC50 of the agonist - the concentration that provokes a response half way between the basal (Bottom) response and the maximal (Top) response. Many log(dose) response curves follow the familiar symmetrical sigmoidal shape. Use a related equation when X values are concentrations or doses. Ĭonsider whether you want to constrain Y0 and/or Ym to fixed values.This equation is used when X values are logarithms of doses or concentrations. After entering data, click Analyze, choose nonlinear regression, choose the panel of growth equations, and choose Logistic growth. Enter time values into X and population values into Y. Conversely, when Y is large, the Gompertz model grows more slowly than the logistic model. But when Y is low, the Gompertz model grows more quickly than the logistic model. Gompertz and logistic models generate curves that are very similar. As Y approaches the maximum, that second term gets smaller so the growth slows. At any given time, the growth rate is proportional to Y(1-Y/YM), where Y is the current population size and YM is the maximum possible size. The logistic model is defined by a linear decrease of the relative growth rate. Logistic growth starts off nearly exponential, and then slows as it reaches the maximum possible population.
